Mastering Data Labeling: The Key to Effective AI Solutions
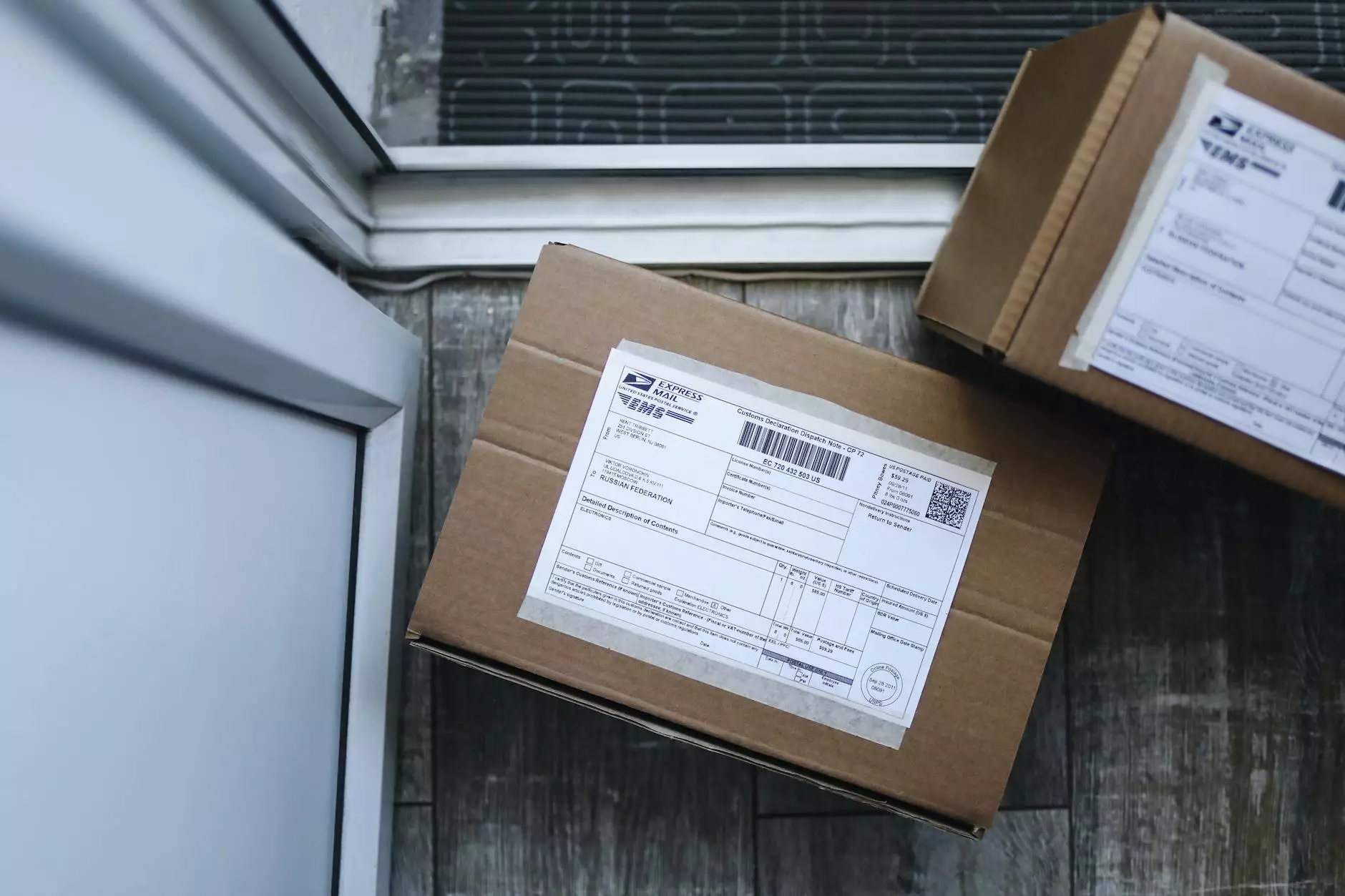
In today's digital landscape, where artificial intelligence (AI) and machine learning are reshaping industries, data labeling has emerged as a foundational pillar for success. As businesses strive to harness the power of AI, understanding the intricacies of data annotation becomes paramount. This article will delve deeply into the significance of data labeling, explore the tools and platforms available, and showcase how KeyLabs.ai can serve as your trusted partner in advancing your data-driven initiatives.
What is Data Labeling?
Data labeling is the process of annotating or tagging data, such as images, audio, text, and videos, to make it comprehensible for machine learning algorithms. In essence, this task transforms raw data into training datasets that AI systems can learn from. The quality and accuracy of data labeling significantly impact the performance of the trained models, making it an essential stage in the AI development process.
The Importance of Data Labeling in Machine Learning
As businesses increasingly implement AI solutions, the necessity for precise data labeling cannot be overstated. Here are several reasons why data labeling is critical:
- Accuracy: Well-labeled data enhances the accuracy of machine learning models, ensuring they can perform their tasks effectively.
- Efficiency: Proper data annotation leads to improved model training times and streamlined processes.
- Scalability: A robust data labeling strategy allows businesses to scale their AI solutions rapidly.
- Customization: Tailored labeling can help organizations build specific AI applications that align with their unique business needs.
Types of Data Labeling
Data labeling can take many forms, depending on the type of data being processed. Below are some common types of data labeling:
1. Image Annotation
Image annotation involves labeling images to train computer vision algorithms. This can include methods such as:
- Bounding Boxes: Drawing boxes around objects in an image.
- Semantic Segmentation: Classifying each pixel in an image.
- Keypoint Annotation: Identifying specific points on objects, such as facial features.
2. Text Annotation
Text annotation involves labeling text data, which is crucial for natural language processing (NLP) tasks. Techniques include:
- Named Entity Recognition (NER): Identifying and categorizing entities in text.
- Sentiment Analysis: Assessing the sentiment expressed in a piece of writing.
- Text Classification: Categorizing documents into predefined classes.
3. Audio Annotation
Audio annotation is essential for training models to understand and process spoken language. It includes:
- Transcription: Converting spoken language into written text.
- Speaker Identification: Labeling different speakers in an audio clip.
- Emotion Detection: Analyzing audio tones to identify emotions.
The Role of Data Annotation Tools in Data Labeling
To facilitate effective data labeling, numerous data annotation tools have been developed. These tools enhance productivity, quality, and consistency in the labeling process. Here are a few prominent data annotation tools:
- Labelbox: A popular choice that provides a user-friendly interface for image and video annotation.
- Prodigy: An annotation tool designed for NLP tasks, allowing quick adjustments to labelling and model training.
- SkyAnnotation: An end-to-end annotation solution that supports various data types.
- KeyLabs.ai: Showcasing advanced capabilities in both image and text annotation, ensuring high accuracy and efficiency.
KeyLabs.ai: Your Partner in Data Labeling
KeyLabs.ai stands out in the realm of data annotation platforms, offering innovative solutions tailored to meet the needs of businesses striving to succeed in their AI initiatives. Here’s why you should consider KeyLabs.ai for your data labeling needs:
- Advanced Technology: KeyLabs.ai employs cutting-edge algorithms to assist in automating data labeling tasks, enhancing efficiency.
- Comprehensive Services: From image and video annotation to NLP and audio labeling, their services span a wide range of applications.
- Quality Assurance: Strict quality control measures ensure that every labeled dataset meets industry standards.
- Flexibility: KeyLabs.ai offers customizable labeling options, allowing you to choose the services that align with your unique requirements.
Challenges in Data Labeling
While data labeling is a critical process, it also comes with its share of challenges:
- Volume of Data: The tremendous amount of data generated daily can overwhelm manual labeling processes.
- Subjectivity: Different annotators may interpret labeling tasks differently, leading to inconsistencies.
- Cost: High-quality data labeling can be costly, especially for large datasets.
- Time-Consuming: Manual labeling can take significant time, delaying project timelines.
Overcoming Data Labeling Challenges
To mitigate the challenges associated with data labeling, businesses can adopt several strategies:
- Utilize Automation: Implementing tools that automate parts of the data labeling process can save time and improve consistency.
- Hire Experts: Bringing in experienced annotators can significantly enhance the quality of labeled data.
- Set Clear Guidelines: Providing comprehensive guidelines for annotators helps to unify understanding and outputs across the team.
- Invest in Quality Control: Establishing robust quality control mechanisms can help catch and correct errors early in the process.
Future Trends in Data Labeling
The landscape of data labeling is evolving, driven by technological advancements and the increasing demand for AI-powered applications. Some trends that are shaping the future of data labeling include:
- AI-Driven Annotation: The use of AI to assist in the labeling process will continue to grow, improving speed and accuracy.
- Collaborative Platforms: More businesses will leverage collaborative platforms that allow teams to work together on large labeling projects.
- Real-Time Data Labeling: As businesses require quicker turnaround times, real-time data labeling solutions will become more prevalent.
- Increased Focus on Quality: As AI applications become critical to business success, maintaining high-quality labeled datasets will be a primary focus.
Conclusion: The Essential Role of Data Labeling in Business Success
In conclusion, data labeling is not just a routine task; it is a crucial element in the success of AI and machine learning strategies. Businesses that recognize the value of high-quality annotated data will position themselves advantageously in an increasingly competitive marketplace. By partnering with a skilled provider like KeyLabs.ai, organizations can ensure their data labeling efforts yield exceptional results that propel their AI initiatives forward.
As technology continues to evolve, staying ahead in the realm of data labeling will be critical for organizations looking to leverage AI for innovation, efficiency, and growth. Embrace the future of data annotation with KeyLabs.ai and unlock new possibilities for your business today!